Document numérique Voix, données
Répertoires d’outils et données en humanités numériques
Olivier Charbonneau 2019-02-28
BigDIVA, Voyant-Tools, Cytoscape, RStudio… et vous, vous utilisez quel outil pour votre projet en humanités numériques ?
La sélection d’un corpus et d’outils d’analyse s’inscrivent dans les choix des chercheurs sur les plans épistémologiques, conceptuels et méthodologiques. L’univers numérique permet à tout-un-chacun de saisir de nouvelles opportunités de développer son propre cadre de recherche, une sorte d’environnement scientifique personnalisé. Et c’est bien là le problème au niveau (trans/multi/inter)disciplinaire : l’opportunité d’innover de par ses choix de sources et d’outils, quitte à rendre son projet unique, introduit divers glissements quant à la reproductibilité et la transférabilité de ses approches.
Dit simplement, nous vivons à une époque offrant des opportunités inégalées de recherche, au point où nous risquons de prendre de vue l’entreprise collective scientifique qui nous motive.
En ce sens, j’applaudis chaleureusement toute initiative de recensement et de description d’outils et de sources pour des projets en humanités numériques. En voici quelques-uns qui sont passés sous mon nez, n’hésitez pas à en ajouter dans la section commentaire de ce billet:
Open Methods du site DARIAH: un répertoire qui recense les méthodes et outils en humanités numériques. Vraiment intéressant comme catalogue pour des démarches intellectuelles.
DiRT:Directory is a registry of digital research tools for scholarly use.
Isidore, Votre assistant de recherche en Sciences Humaines et Sociales (sources, documents, sujets), surtout de France
De nos amies en Australie, le site HuNi pour les données artistiques et culturelles
Document numérique Lettres
Avoir la mesure des mots (lecture de Hermeutica de Rockwell & Sinclair, MIT Press 2016)
Olivier Charbonneau 2017-08-23
Suite à mon exploration de l’outil Voyant-tools pour l’analyse textuelle, la Société canadienne des humanités numériques a décerné son prix annuel à Stéfan Sinclair, Professeur à McGill et directeur du centre de recherche sur les humanités numériques, et À Geoffrey Rockwell, professeur de philosophie à l’University of Alberta, pour leur outil Voyant-tools ainsi que leur livre décrivant leur parcours intellectuel intitulé: Hermeneutica : computer-assisted interpretation in the humanities. Je me suis empressé d’emprunter et de lire leur monographie afin de mieux comprendre l’outil qu’ils ont créé…
Les auteurs offrent une réflexion sur leur démarche intellectuelle dans le recours à des outils technologiques pour l’analyse textuelle sourtout dans le domaine des études littéraires. Voici quelques passages qui ont retenu mon attention. Par ailleurs, je cite deux autres livres à la toute fin de ce billet – ils concernent eux aussi le domaine (surtout le livre de Holsti, qui ne figure pas dans la bibliographie de Rockwell & Sinclair mais qui traite d’un sujet très proche).
Je crois que le travail de Rockwell & Sinclair dans Hermeneutica permet de mieux comprendre comment le domaine des études littéraires s’est approprié l’outil informatique. J’espère m’en inspirer largement dans l’analyse empirique et informatique du droit… Selon les auteurs,
This book, to be clear, is not about what we can learn from corpus linguistics or computational linguistics. Rather, it is rooted in the traditions of computer-assisted text analysis in literary studies and other interpretive disciplines and the more hermeneutical ends of philosophy and history. It is about that tradition as much as it is about what you can do with hermeneutical things. Insofar as the tradition of literary text analysis has evolved in parallel with computational linguistics without building on it, we feel justified in leaving that for another project.
The use of interpretive tools in the social sciences is also outside the purview of this book. There is a tradition of qualitative techniques and tools for analyzing interviews or other texts of interest to social scientists. This is similar to literary text analysis, in that it uses computers to interpret texts, but the goals and methods are different. Atlas.ti, NVivo, and similar tools help social scientists with content analysis for social scientific aims. They are not meant to help understand the texts themselves so much as to help understand the phenomena of which texts such as interview transcripts are evidence. Again, as with linguistic analysis, we could learn a lot from such
(p. 19)
tools and traditions of research practice, but they are beyond the scope of this book. (p. 20)
En citant les travaux de Smith, Rockwell & Sinclair tentent de développer une approche théorique basée sur une approche itérative de l’interrogation d’un corpus (p.86-7). Smith a précédé Rockwell & Sinclair de quelques décennies mais fut confronté à la même problématique qu’eux: comment développer une approche méthodologique face à la montagne de données, statistiques, mesures, et autres calculs possibles sur un corpos précis.
In his article “Computer Criticism” Smith completes the move from imagery, as it is manifest in computer visualizations of various kinds, to a critical theory that opens the way for computational methods. What makes this hermeneutical circle even more interesting is that the aesthetic theory voiced by Stephen Dedalus nicely captures the encounter with the visualization. Dedalus has developed an idea from the medieval philosopher Thomas Aquinas. The encounter with art, Dedalus argues, passes through three phases:
1. Integritas: First, the aesthetic image (visual, audible, or literate) is encountered in its wholeness as set off from the rest of the visible universe.
[…]
2. Consonantia: Then one apprehends the harmony of the whole and the parts.
(p. 88)
[…]
3. Claritas: And finally one sees the work in its particular radiance as a unique thing, bounded from the rest but with harmonious parts. […]
These three phases or this aesthetic encounter make a pattern that we can use for the interpretive encounter. The sequence might be something like the following steps, the first two having been outlined in chapter 2 and the third covering how results are returned to you (the subject of this chapter).
Demarcation First, one has to identify and demarcate the text (or corpus) one is going to interpret. In that choice one is defining the boundary of a work or collection from the rest for the purposes of interpretation. Pragmatically this takes the form of choosing a digital text and preparing it for study.
Analysis Second, one breaks the whole into parts and studies how those parts contribute to the form of the whole. Smith, in “Computer Criticism,” suggests that this is where the computer can assist in interpretation. Stephen Dedalus summarizes this as analyzing “according to its form.” 18 The computer can automate the analysis of a text into smaller units (tokens). Even individual words can be identified and be used as the unit for analysis.
Synthesis Third, there is a synthesis of the analytical insights and interpretive moves into a new form that attempts to explain the particular art
of the work being studied. That is the synthesis into visualizations and then into an essay; a new work that attempts to clarify the interpreted work. These syntheses are hermeneutica.
When we return later to theorize computer-assisted interpretation, we will look more closely at Smith’s structuralist critical theory, the weakest part of his triptych of illustrative essay, theory, and tools.
(références pour ce passage)
Smith , J. B. Image and imagery in Joyce’s Portrait: A computer-assisted analysis . In Directions in Literary Criticism: Contemporary Approaches to Literature, ed. S. Weintraub and P. Young . University Park : Pennsylvania State University Press , 1973 .
Smith , J. B. 1978 . Computer criticism. Style 12 ( 4 ): 326 – 356 .
Smith , J. B. 1984 . A new environment for literary analysis. Perspectives in Computing 4 ( 2/3 ): 20 – 31 .
Smith , J. B. 1985 . Arras User’s Manual. TextLab Report TR85-036, Department of Computer Science, University of North Carolina, Chapel Hill.
(Important: Stephen Dedalus est l’aterego littéraire de James Joyce, l’auteur qu’analyse Smith.)
Les auteurs considèrent que l’accessibilité accrue à des corpus numériques et aux outils pour les analyser introduisent des mutations dans leur discupline:
Big data has been trumpeted as the next big thing made possible by the explosion of digital data about us, to which we contribute. As we write this, big data probably is at the high point in the Hype Cycle —there are inflated expectations as to how it will transform business, government, and the academy, and soon there are likely to be more sober evaluations. Drawing on Mayer-Schönberger and Cukier’s book Big Data, we characterize how
big data changes methods as follows:
From samples to comprehensive data In the humanities and the social sciences, traditionally we either focus on works that are considered distinctive or sample larger phenomena. Big data draws on relatively complete datasets, often created for entirely different purposes, and uses them for new research. These datasets are comprehensive in a way that sampling isn’t, and in a way that can change the types of questions asked and can change people’s confidence in the answers.
(p. 125)
From purposeful data to messy and repurposed data Traditionally, the data used in research are carefully gathered and curated for that research. With big data, we repurpose messy data from other sources that weren’t structured for research and we aggregate data from multiple sources. This raises ethical issues when people’s information is used for purposes they didn’t consent to; it also means that we can extract novel insights from old data, which is why preservation of data is important.
From causation to correlation Big data typically can’t be used to prove causal links between phenomena the way experiments can. Instead, big data is used to show correlations. Big data is being pitched as an opportunity to reuse data to explore for new insights in the form of correlations. There are even techniques that can comb through datasets to identify all the statistically significant correlations that could be of research interest.
What Does This Mean for the Humanities?
What does this mean for the humanities in general and the Digital Humanities in particular? Well, there are obvious privacy issues, ethical issues, and governance issues that call for attention. The liberal arts are supposed to prepare free ( liber) people for citizenship and participation in democratic community. Big data is now one way citizens are managed both by industry and by government. 25 The liberal arts clearly have a role in studying and teaching about big data. To get a conversation of the phenomenon going, the researchers danah boyd and Kate Crawford (2012) offer six provocative statements:
1. Big Data changes the definition of knowledge.
2. Claims to objectivity and accuracy are misleading.
3. Bigger data are not always better data.
4. Taken out of context, Big Data loses its meaning.
5. Just because it is accessible does not make it ethical.
6. Limited access to Big Data creates new digital divides.
Following boyd and Crawford, one way to think about big data is to look at the pragmatics of how working with large amounts of data changes knowledge. A particularly Digital Humanities way to do this is to think through experimenting with the analysis of large datasets. “Thinking through” is an approach of understanding a phenomenon (thinking about it) through the practices of making, experimenting, and fiddling. It is one of the ways the Digital Humanities can contribute to the larger dialogue of the humanities through what Tito Orlandi and later Willard McCarty called
(p. 126)
modeling. We create models of systems and learn through the iterative making and reflecting on the models—especially when they fail, as they usually do. The false positives that our hermeneutica throw up tell us about the limitations of our models and hence about the limitations of the theories of knowledge on which they are based.
Ainsi, (p. 128-130)
What Can We Do with Big Data Analytics?
At this point, let us take a quick tour through some of the opportunities and risks for Big Data in the Digital Humanities.
Filtering and subsetting We begin with a basic operation comparable to what intelligence services do with Information in Motion: filtering big data to get useful subsets that can then be studied by other, possibly traditional techniques (e.g., reading). The Cornell Web Lab, which alas was closed in 2011, was working with the Internet Archive to build a system that would enable social scientists (and humanists) to extract subsets from the archive. The dearth of resources that allow for navigation between large databases of content (among them the Gutenberg Project of digital texts) to specialized environments for reading and analysis should be noted here.
Enrichment “Enrichment” is a general term for adding value. In the context of big data, researchers are developing ways to enrich large corpora automatically using the knowledge in big data. In “What Do You Do with a Million Books?” (the 2006 article that first prompted many of us to begin thinking about what big data meant in the humanities), Greg Crane wrote eloquently about what we now can do to enrich big data to make it more useful. Crane has made the further point that simply providing translation enrichment could provide us with a platform for a dialogue among civilizations.
Sequence alignment Sequence alignment can be adapted from bioinformatics for the purpose of following passages through time. This is the research equivalent to what plagiarism detectors do with students’ papers. They look at sequences of text to see whether similar passages can be found in other texts, contemporary or not. Horton, Olsen, and Roe (2010) described work they had done with the ARTFL textbase to track how passages in an influential twentieth-century history by Paul Hazard had been cribbed from a seventeenth-century text. The point is not that Hazard was a lazy note taker, but that one can follow the expression of ideas across writers when one has them in digital form.
Diachronic analysis Diachronic analysis is using data sets to study change over time. Although the use of large diachronic databases dates back to
(p. 129)
the Trésor de la langue française (TLF) and the Thesaurus Linguae Grecae (TLG) projects, diachronic analysis got a lot of attention after Google made its Ngram Viewer available and after Jean-Baptiste Michel and colleagues published their paper “On Quantitative Analysis of Culture Using Millions of Digitized Books” (2010). There are dangers to using Ngrams (or phrases of words) to follow ideas when the very words have changed in meaning, orthography, and use over time, but the Ngram Viewer is nonetheless a powerful tool for testing change in language over time (and its search-and-graph approach makes it accessible to a broad public).
Classification and clustering Classification and clustering comprise a large family of techniques that go under various names and can be used to explore data. Classification and clustering techniques typically workon large collections of documents and allow us to automatically classify the genre of a work or to ask the computer to generate clusters according to specific features and see if they correspond to existing classifications. A related technique, Topic Modeling, identifies clusters of words that could be the major “topics” (distinctive terms that co-occur) of a large collection. An example was given in the previous chapter, where we used correspondence analysis to identify clusters of key words and years in the Humanist archives.
Social network analysis Social network analysis (SNA) involves identifying people and other entities and then analyzing how they are linked in the data. It is popular both in the intelligence community and in the social sciences. SNA techniques can graph a network of people to show how they are connected and to what degree they are connected. In the social sciences, the data underlying a network typically are gathered manually. One might for example interview members of a community in order to understand the network of relationships in that community. The resulting data about the links between people can be visualized or queried by computer. These techniques can be applied in the humanities when one wants to track the connections between characters in a work (Moretti 2013), or the connections between correspondents in a collection of letters or places mentioned in a play. Just as an ethnographer might formally document relationships between people, a historian might be interested in determining who lived in Athens when Socrates was martyred and how those people were connected. Large collectionsof church records, letters, and other documents now allow us to study social networks of the past. Thanks to a new family of techniques that can recognize the names of people, organizations, or places in a text, it is
(p. 130)
now possible to extract named entity data automatically from large text collections, as the Voyant tool called RezoViz does.
Self-tracking Self-tracking is the application of big data to your life. You can gather big data about yourself. Personal surveillance technologies allow you to gather lots of data about where you go and who you correspond with, and to then analyze it. The idea is to “know thyself” better and in more detail (or perhaps just to remind you to exercise more). Though results of self-tracking may not seem to be big data relative to the datasets gathered by the NSA, they can accumulate to the point where one must use mining tools to make sense of it. A fascinating early project in life tracking was Lifestream, started in the 1990s as a project at Yale by Eric Freeman and David Gelernter, which introduced interesting interface ideas about how information could be organized according to the chronological stream of life. 31 Gordon Bell has a project at Microsoft called MyLifeBits that has led to a commercially available camera, the Vicon Revue; you hang it around your neck, and it shoots pictures all day to frame bits of your life. 32 Recently Stephen Wolfram, of Mathematica fame, has posted an interesting blog essay on what he has learned by analyzing data he gathered about his activities—an ongoing process that, he argues, contributes to his self-awareness. 33 Many different tools are now available to enable the rest of us to gather, analyze, and share data about ourselves—especially data on our physical fitness data. (Among those tools are the fitbit, the Nike+ line, 34 and now the Apple Watch.)
Les auteurs (p. 128) proposent les lectures suivantes pour aller plus loin:
Jockers , M. L. 2013 . Macroanalysis: Digital Methods and Literary History. Urbana : University of Illinois Press .
Moretti , F. 2007 . Graphs, Maps, Trees: Abstract Models for Literary History. London : Verso .
Moretti , F. 2013 a. The end of the beginning: A reply to Christopher Prendergast . In Moretti, Distant Reading. London : Verso ,
Moretti , F. 2013 b. Network theory, plot analysis . In Moretti, Distant Reading . London : Verso .
L’analyse de données massives et textuelles amène certains écueils.
The problem is that when you analyze a lot of data you get a lot of false hits or false positives. The messier the data, the more false hits you get. The subtler the questions asked of the data, the more nuanced and even misleading the answers are likely to be. When you use Google to search for someone with a common name, such as John Smith, with big data you can get overwhelmingly big and disappointingly irrelevant results—result sets that are too big for you to go through to find the answer, and therefore not useful. (p. 131)
Par ailleurs,
A second problem with analysis of big data is the dependence on correlation. It doesn’t tell you about what causes what; it tells you only what correlates with what (Mayer-Schönberger and Cukier 2013, chapter 4). With enough data one can get spurious correlations, as there is always something that has the same statistical profile as the phenomenon you are studying. This is the machine equivalent to apophenia, the human tendency to see patterns everywhere, which is akin to what Umberto Eco explores in Interpretation and Overinterpretation (1992).
The dependence on correlations has interesting implications. In 2008, Chris Anderson, the editor in chief of Wired, announced “the end of theory,” by which he meant the end of scientific theory. He argued that with lots of data we don’t have to start with a theory and then gather data to test it. We can now use statistical techniques to explore data for correlations with which to explain the world without theory. Proving causation through the scientific method is now being surpassed by exploratory practices that make big science seem a lot more like the humanities. It remains to be seen how big data might change theorizing in the humanities. Could lots of exploratory results overwhelm or trivialize theory? What new theories are needed for working with lots of data?
A related problem is “model drift” or “concept drift,” which is what happens when the data change but the analytical model doesn’t. […] The fact that people’s use of language changes over time is a major problem for humanities projects that use historical data. People don’t use certain words today the same way they used those words in the past, and that’s a problem for text (p. 133) mining that depends on words. If you are modeling concepts over time, the change in language has to be accounted for in the model—an accounting that raises a variety of interpretive questions. Does a phrase that you are tracking have the same meaning it had in the past, or has its meaning evolved?
(p. 132-3)
Eco , U. 1992 . Interpretation and Overinterpretation. Cambridge University Press .
Mayer-Schönberger , V. , and K. Cukier . 2013 . Big Data: A Revolution That Will Transform How We Live, Work, and Think. New York : Houghton Mifflin Harcourt
Ainsi, les auteur concluent (p. 133-4)
Similar questions about cost effectiveness should be asked, and are being asked, about text analysis and mining in the humanities. Does text mining of large corpora provide real insight? There are two types of questions that we need to keep always in mind in analysis, especially in regard to largescale analysis:
• Are the methods and their application sound, and can they be tested by alternative methods (or at the very least, are we sufficiently circumspect about the data and methodologies)? In large-scale text analysis or “distant reading,” it is easy to misapply an algorithm and generate a false result. It is also easy to overinterpret results. Since at the scale of big data one can’t confirm a result by re-reading the sources, it is important to
confirm results in various ways. There is a temptation to use the size of the data to legitimize not testing results by reading, but there are always way to test results. Jockers (2013) shows us how one should always be (p. 134) sceptical of results and develop ways of testing results even if one can’t
test them by reading.
• Are the results worth the effort? Obviously, people who engage in text analysis and in data mining believe that the results are interesting enough to justify continuing, but at an individual level and at a disciplinary level we need to ask whether these methods are worth the resources. Computational
analysis is expensive not so much in terms of computing (of which there is an ample supply thanks to high-performance computing initiatives) as in terms of the human effort required to do it well. Is computational analysis worth the training, the negotiating of resources, and the programming it requires? Individuals at Voyant workshops ask this question in various ways. Also important are the disciplinary discussions taking place in departments and faculties that are deciding whether to hire in the Digital Humanities and whether to run courses in analytics. Hermeneutica can help those departments and faculties to “kick the tires” of text analysis as a pragmatic way of testing analytical methods in general.
Les auteurs offrent un exemple de leur cru des écueils de l’analyse de données massives et textuelles.
We have our own story about too many results—a story that points out another form of false positive in which the falsity is more that of a false friend. In May of 2010 we ran a workshop on
High-Performance Computing (HPC) and the Digital Humanities at the University of Alberta. We brought together a number of teams with HPC support so they could prototype HPC applications in the humanities. One team adapted Patrick Juola’s
idea for a Conjecturator (Juola and Bernola 2009) so that it could run on one of the University of Alberta’s HPC machines. The Conjecturator is a data-mining tool that can generate a nearly infinite number of conjectures about a big dataset and then test them to see if they are statistically interesting.
The opposite of a “finding” tool that shows only what you ask for, it generates statistically tested assertions about your dataset that could be
studied more closely. The assertions take this form:
Feature A appears more/less often
in the group of texts B than in group C
that are distinguished by structural feature D
Citant:
Juola, Patrick, Bernola, Ashley (2009). ‘Conjecture Generation in the Digital Humanities’. Proc. DH-2009. 2009
Les auteurs indiquent que cette approche, lorsqu’appliquée au corpus de tous les livres publiés en 1850 génère plus de 87000 affirmations positives!! Ils pointent vers la fin de la théorisation scientifique classique (p. 132 – citant Chris Anderson qui, en 2008, a annoncé la fin de la théorisation scientifique). Donc, quoi faire avec 87000 théories?
• Many of the results are trivial. They may be statistically valid, but many nonetheless are uninteresting to humans.
• Many of the results are related. You might find that there is a set of related assertions about shirts over many decades.
• The results are hard to interpret in the dialogical sense. You may find a really interesting assertion but have no way to test it, follow it, or unpack it. The Conjecturator is not usefully connected to a full-textanalysis
environment in which one could explore an interesting assertion. As always, the trajectory from the very atomistic level (the narrow and specific assertions) to larger, more significant aspects is unmarked and hazardous, though not without potential rewards. (p. 135)
Vers une théorie modèle
Les auteurs (p. 152) indiquent que les travaux de Busa, avec l’aide de Tasman qui travaille pour IBM sont un pount de départ pertinent pour l’émergence des humanités numériques en études littéraires:
These are some of the first theoretical reflections on text analysis by a developer. Though they aren’t the earliest such reflections, Busa’s ideas and his project are generally considered major initial influences in DH. 10 His essay positioned concordances as tools for uncovering and recovering the meanings of words in their original contexts. Busa is in a philological tradition that prizes the recovery of the historical context of language. To interpret (p. 153) a text, one must reconstitute the conceptual system of its author and its author’s context independent of the text.
A concordance refocuses the philological reader on the language. It structures a different type of reading: one in which a reader can see how a term is used across a writer’s works, rather than following the narrative or logic of an individual work. A concordance breaks the train of thought; it is an instrument for seeing the text differently. One looks across texts rather then through a text. The instrument, by its very nature, rearranges the text by showing and hiding (showing occurrences of key words and their context grouped together and hiding the text in its original sequence) so as to support a different reading.
Busa , R. 1980 . The annals of humanities computing: The Index Thomisticus. Computers and the Humanities 14 ( 2 ): 83 – 90 .
Tasman , P. 1957 Literary data processing. IBM Journal of Research and Development 1 ( 3 ): 249 – 256 .
J’aime particulièrement cette idée selon laquelle: One looks across texts rather then through a text. The instrument, by its very nature, rearranges the text by showing and hiding (p. 153)
En poursuivant leur survol des textes fondateurs, les auteurs proposent ceci sur Smith 1978 (précité), à la page 156:
Smith reminds us that the term “computer criticism” is potentially misleading, insofar as the human interpreter, not the computer, does the criticism. Smith insists that “the computer is simply amplifying the critic’s powers of perception and recall in concert with conventional perspectives.” [Smith 1978, p327] It would be more accurate to call his theory one of “computer-assisted criticism,” in a tradition of thinking of the computer as extending hermeneutical abilities. According to Smith, “the full implications of regarding the literary work as a sequence of signs, as a material object, that is “waiting” to be characterized by external models or systems, have to be realized.” [ibid] The core of Smith’s theory, however, comes from an insight into the particular materiality of the electronic text. As we pointed out in chapter 2, computers see a text not as a book with a binding, pages, ink marks, and coffee stains, but as a sequence of characters. The electronic text is a formalization of an idea about what the text is, and that formalization translates one material form into another. It is just another edition in a history of productions and consumption of editions.
The computer can help us to identify, compare, and study a document’s formal structures or those fitted to it. The process of text analysis, with its computational tools and techniques, is as much about understanding our interpretations of a text, by formalizing them, as it is about the text itself. Smith is not proposing that the computer can uncover some secret structure in the text so much as that it can help us understand the theoretical
structures we want to fit to the text.
An example of a structure interpreted onto the text is a theme. Here is what Smith wrote about mapping themes onto Joyce’s prose by searching
for collections of related words:
The concept of distribution is a diachronic, “horizontal” concept of structure that characterizes patterns along one of the vertical strata described earlier; a different concept of form or structure is the collection of synchronic relations among a number of such distributions. Synchronic patterns of interrelation are, essentially, patterns of co-occurrence. [Citant Smith 1978, p. 339]
In “Computer Criticism” (1978) and “A New Environment for Literary Analysis” (1984) Smith talks about following a “fire” theme; in “Image and Imagery in Joyce’s Portrait” (1973) he gives an example of how he studies such thematic structures. Smith describes a mental model of the text as vertical layers or columns. Imagine all the words in the text as the leftmost base column, with one word per line. Then imagine a column that marks which words belong to the fire theme. That layer could be plotted horizontally in a distribution graph. If one has a number of these structural columns, then one can also study the co-occurrence of themes across rows. Do certain themes appear in the same paragraphs, for example?
Smith anticipated text-mining research methods that are only now coming into use. He describes using Principal Component Analysis (PCA) to identify themes co-occurring synchronically, then cites examples of state diagrams and CGAMS that can present 3D models of the themes in a text. The visualizations are graphical presentations of structural models can be used to explore themes and their relations. [Smith 1978 p.343]
In the late 1970s, Smith was trying to find visual models that could be woven into research practices. He called some of his methods Computer Generated Analogues of Mental Structure (CGAMS), drawing our attention to how he was trying to think through the cognitive practices of literary study that might be enabled by the computer. Like Perry’s model railroad, CGAMS provide a model theory that has explanatory and scalable power. The visualizations model a text in a way that can be explored, and they act as examples that a computer-assisted critic can use to model his or her own interpretations.
Les auteurs poursuivent en précisant que Smith a lié ses efforts à l’analyse littéraire en passant par les travaux de Rolland Barthes et surtout, le structuralisme (Barthes , R. 1972 . The Structuralist Activity: Critical Essays. Evanston : Northwestern University Press), ainsi:
structuralism should not be seen as a discovering of some inherent or essential structure, but as an activity of composition (p. 157)
[…] citation de Barthes 1972, pp. 214-5 & Référence ibid, p. 216
Ainsi:
That the computer forces one to formally define any text and any method makes this structuralist understanding of interpretation even more attractive as a way of understanding computer-assisted criticism.
The computer can help us with the activities of dissection and composition when what we want to study has been represented electronically. 27 The computer can analyze the text and then synthesize new simulacra for interpretation that are invested with the meanings that we (both the “we” of the developers of the tools and the “we” of users issuing queries) bring. (p. 158)
[À la note 27, les auteurs citent Rockwell , G. 2001 . The visual concordance: The design of Eye-ConTact. Text Technology 10 ( 1 ): 73 – 86. Rockwell utilise l’analogie du monstre pour l’analyse littéraire: comme Frankenstein qui est composé de divers morceaux ]
Les auteurs (p. 162) proposent que deux classes de théories sont pertinentes dans les humanités numériques: les instruments et les modèles.
À propos des instruments:
Certain instruments are theories of interpretation. An instrument implements a theory of interpreting the phenomenon it was designed to bring into view. It orients the user toward certain features in the phenomenon and away from others. The designer made the choice of what to show and what to hide. The user may tune it, but the instrument is, in effect, saying “This is important; that is not.” As Margaret Masterman wrote in “The Intellect’s New Eye” (1962), “the potential capacity of the digital computer to process nonnumerical data in novel ways—that capacity the surface of which has hardly been scratched as yet—is so great as to make of it the telescope of the mind.” (p. 162)
Citant
Masterman , M. 1962 . The intellect’s new eye . In Freeing the Mind: Articles and Letters from the Times Literary Supplement during March–June, 1962 . London : Times .
Quant aux modèles,
Thus, the things of the Digital Humanities are all models that are formalized interpretations of cultural objects. Even a process as apparently trivial as digitizing involves choices that foreground things that we formerly took for granted, forcing us to theorize at least to the point of being able to formalize. In order to be able to know what we are digitizing and how to do so, we need to develop a hermeneutical theory of things.
Through the process of digitizing we end up with three types of models: a theoretical model of the problem or cultural phenomenon; a working model of an instance of the phenomenon based on the first; and a model of the process whereby we will get the second, which may be semi-automated in code. The first is a model that is closer to what we mean by a hermeneutical theory. The second is the software, such as the e-text, that instantiates the theory to a greater or a lesser extent. The third is a model of the processing of the second, and that is what can be captured in practices and in tools (whether digitizing tools, analytical tools, or presentational tools). P. 163)
Call Number |
AZ 186 R63 2016 |
|
Title |
Hermeneutica : computer-assisted interpretation in the humanities / Geoffrey Rockwell and Stéfan Sinclair. |
|
Description |
viii, 246 pages : illustrations ; 24 cm. |
Bibliography |
Includes bibliographical references (pages [227]-239) and index. |
Contents |
Introduction: Correcting method — The measured words : how computers analyze texts — From the concordance to ubiquitous analytics — First interlude: The swallow flies swiftly through : an analysis of Humanist — There’s a toy in my essay : problems with the rhetoric of text analysis — Second interlude: Now analyze that! : comparing the discourse on race — False positives : opportunities and dangers in big text analysis — Third interlude: Name games : analyzing game studies — A model theory : thinking-through hermeneutical things — Final interlude: The artifice of dialogue : thinking-through scepticism in Hume’s dialogues — Conclusion: Agile hermeneutics and the conversation of the humanities. |
Scope and content |
« With increasing interest being shown in participatory research models, whether it be Wikipedia, World of Warcraft, participatory writing (like Montfort et al’s 10 Print or Laurel et al’s Design Research) or the more traditional communal research cultures of the arts collective or engineering lab, the Humanities is increasingly relying on computational tools to do the ‘heavy lifting’ necessary to process all of this information. Hermeneuti.ca, as its name implies, is about hermeneutical things–the computing tools of research that are usually hidden–how to use them, and how they are interpretative objects to be understood. Hermeneuti.ca is both a book and also a web site (http://hermeneuti.ca) that shows the interactive text analysis tools woven into the book. Essentially, Hermeneuti.ca is both a text about computer-assisted methods and a collection of analytical tools called Voyant (http://voyant-tools.org) that instantiate the authors ideas. While there is a definitely an emphasis on classic Digital Humanities work (corpus analysis, information retrieval, etc.), there is also a focus on the development of software as part of a project of knowledge that encompasses the idea of software as an active part of knowledge production that brings this book into the Software Studies series »–Provided by publisher. |
Subject Heading |
Humanities — Research. |
Digital humanities. |
Humanities — Methodology. |
Alternate Author |
Sinclair, Stéfan, author. |
ISBN |
9780262034357 hardcover ; alkaline paper |
0262034352 hardcover ; alkaline paper |
|
Call Number |
QA 76.76 H94B37 2013 |
|
Title |
Memory machines : the evolution of hypertext / Belinda Barnet ; with a foreword by Stuart Moulthrop. |
|
Description |
xxvi, 166 pages ; 24 cm. |
Series |
Anthem scholarship in the digital age. |
|
Anthem scholarship in the digital age. |
Bibliography |
Includes bibliographical references and index. |
Contents |
Foreword: To Mandelbrot in Heaven / Stuart Moulthrop — Technical Evolution — Memex as an Image of Potentiality — Augmenting the Intellect : NLS — The Magical Place of Literary Memory : Xanadu — Seeing and Making Connections : HES and FRESS — Machine-Enhanced (Re)minding : Development of Storyspace. |
Subject Heading |
Hypertext systems — History. |
ISBN |
9780857280602 (hardcover : alkaline paper) |
|
0857280600 (hardcover : alkaline paper) |
|
Title |
Content analysis for the social sciences and humanities / Ole R. Holsti. — |
|
Publisher |
Reading, Mass. : Addison-Wesley Pub. Co., [1969] |
|
Document numérique Droit Test
Cadres juridiques des documents numériques
Olivier Charbonneau 2017-06-22
Je suis retombé par hazard sur le livre de Mélanie de Dulong de Rosnay intitulé Les Golems du numérique : Droit d’auteur et Lex Electronica et je me suis mis à chercher une liste exhaustive des cadres juridiques applicables aux documents numériques et j’ai déterré deux acétates d’une présentation que j’ai fait dans le cadre du cours de Réjean Savard en 2010.
Dans la première, Gowers (2006 , p. 13) propose une ontologie simplifiée du champ d’application de la propriété intellectuelle à la connaissance.
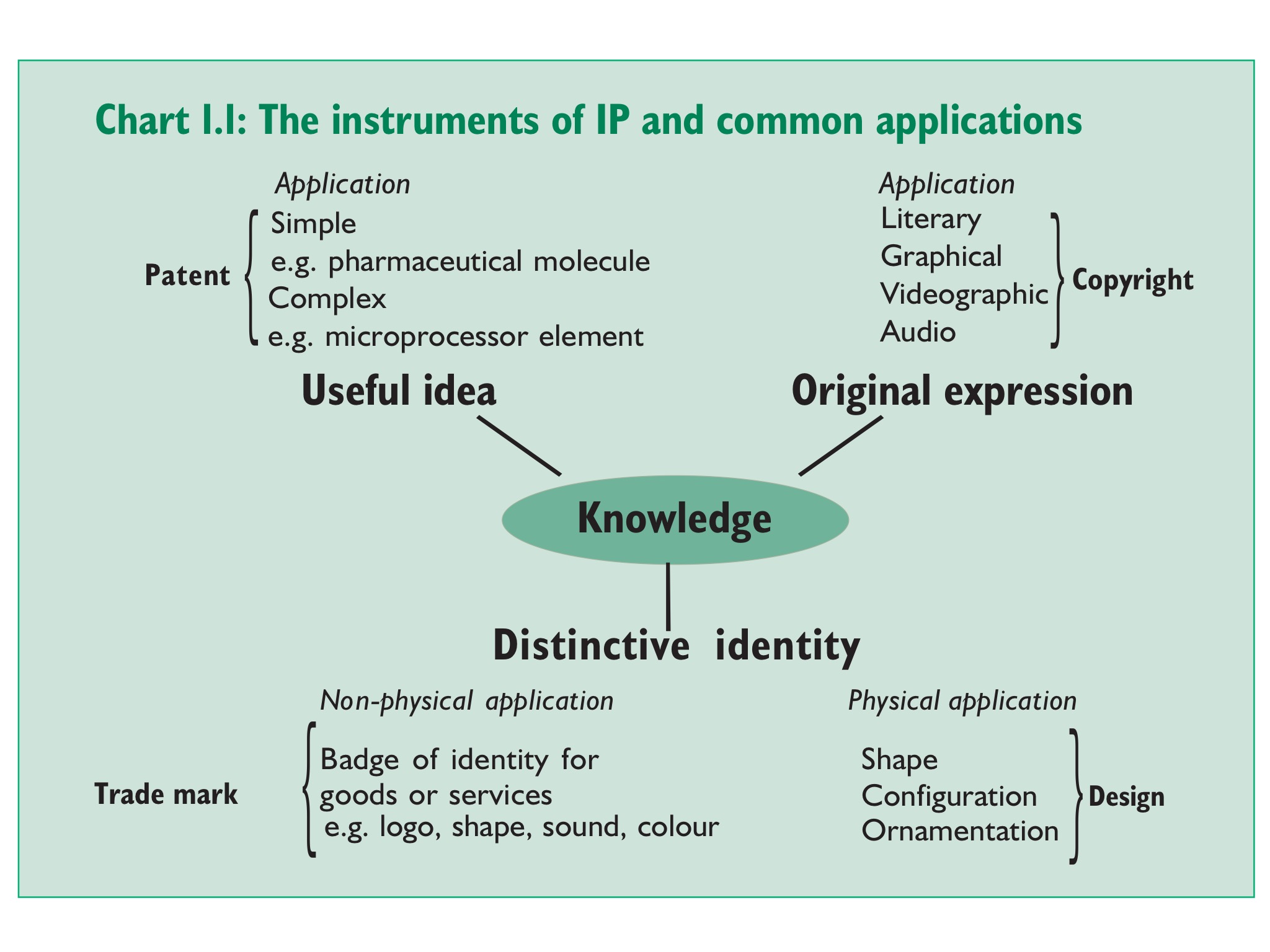
Dans la seconde, je tente de nommer tous les cadres juridiques applicables aux sciences de l’information (mon intention en 2010).

Maintenant, je tente de recenser tous les cadres juridiques applicables aud documents numériques. En ce qui concerne le droit, ce que le document contient déterminera le cadres juridiques applicable. Ainsi:
1. Si le document contient de la connaissance, il se peut que le droit de la propriété intellectuelle s’applique (c.f. : l’image de Gowers ci-haut) selon le cas: droit d’auteur, brevet, marque de commerce, design industriel, etc. Il se peut que les lois sur le statut d’artiste, les bibliothèques/archives nationales, le développement de l’industrie du livre, la liberté d’expression, la diffamation et le secret industriel soient à considerer.
2. Si le document provient d’une instance gouvernementale ou d’une organisation détenant des informations publiques (certaines données financières d’entreprises cotées en bourse; débiteurs de l’État; récipiendaires d’une subvention…), les lois sur l’accès aux documents publics s’appliquent ainsi que la loi sur le cadre juridique des TI selon le cas.
3. Si le document inclut des éléments concernant des personnes physiques, les lois sur la gestion des renseignements personnels, la vie privée, l’anonymat ou le droit à l’image s’appliquent.
4. Les marchés (analyse économique du droit), plateformes (lex électronica: la gouvernance par la technologie) ou organisations où les documents numériques sont créés ou échangés, ainsi que les contrats qui y sont négociés (e.g.: les moyens privés de bâtir un cadre juridique), peuvent favoriser l’émergence d’un système juridique privé ou non-régalien : réglementation, compétition, standards, gouvernance, normes, subventions de l’État, contrats types, métadonnes juridiques.
Commerce et Compagnies Document numérique Voix, données
Lecture de Weapons of Math Destruction (Cathy O’Neil, Crown Publishers, 2016)
Olivier Charbonneau 2016-12-08
Et si les algorithmes étaient en train de détruire notre société ? Cette surprenante prémisse est le point de départ de Cathy O’Neil dans son récent livre sur ce qu’elle nomme les weapons of math destruction ou WMD : les armes de destruction mathématiques. J’ai entendu parlé de l’ouvrage de O’Neil via la baladodiffision Sparc de Nora Young, diffusée à la mi-octobre, une émission web de la Canadian Broadcasting Corporation (CBC) sur les technologies.
Dans ce livre, l’auteure relate une série d’anecdotes soit personnelles, soit plus générales, groupées par thématiques. Ayant travaillé dans le domaine de la haute finance et de l’analyse des données massives en marketing numérique, O’Neil possède une expérience de terrain sur le recours au modèles statistiques et probabilistes appliqués à divers domaines économiques ou sociaux: les admissions à l’enseignement supérieur, le marketing web, la justice, la dotation et la gestion d’horaire en entreprise, l’attribution de cotes de crédit et le profilage pour le vote. Tous ces exemples illustrent comment les données massives, combinées à des processus algorithmiques, mènent à la codification de préjudices où les iniquités systémiques pénètrent dans la chambre de résonance du numérique (p. 3-4).
La première étape pour concevoir un algorithme consiste à bâtir un modèle : une représentation abstraite du monde réel, de laquelle nous pouvons obtenir or extraire des données. Il devient ainsi possible de prévoir ou prédire notre fiabilité, notre potentiel ou notre valeur relative par rapport à ces mesures (p. 4) afin d’optimiser ledit système social ou économique. Beaucoup des problèmes selon O’Neil découlent d’une césure entre le monde et son modèle, il manque un élément de rétroaction (feedback, p. 6) afin de raffiner les calculs et indices numériques issus du modèle. Les exceptions génèrent des cas erratiques et troublent l’harmonie du système: « [WMD] define their own reality and use it to justify their results. This model is self-perpetuating, highly destructive – and very common. […] Instead of searching for the truth, the score comes to embody it » (p. 7).
Un des problème majeur selon O’Neil découle du secret qui entoure les modèles, les données et les algorithmes qui y sont appliqués. En plus d’être camouflée par les mathématiques souvent complexes et inaccessibles (p. 7), les assises sur lesquelles elles reposent ne sont ni testées, ni questionnées. Les pauvres y sont des proies faciles : puisqu’un algorithme se déploie rapidement et à faible coût, il est plus simple de traiter des masses de dossiers, tandis que les personnes plus fortunées reçoivent un traitement plus personnalisé. Par ailleurs, les algorithmes sont des secrets corporatifs jalousement gardés, ce qui complexifie d’avantage une critique sociale constructive (p.8).
Par ailleurs, une fois que l’algorithme est établi et que son score fait autorité, les humains tombant dans ses griffes sont tenus à un fardeau de preuve beaucoup plus élevé pour faire modifier leur score. Si l’algorithme est secret et obscur, son score est accepté et il est difficile de le renverser (p. 10). Il s’agit de dommages collatéraux (p, 13).
Ayant mis la table dans son chapitre introductif (que la mathématicienne n’a pas numéroté et serait le chapitre « 0 »), O’Neil présente les éléments constituant les « bombes » mathématiques, spécifiquement ce q’est un modèle. Elle emploie l’exemple des statistiques sportives, notamment pour le Baseball, puisqu’un livre récent a traité des analyses mathématiques desdites statistiques et fut porté à l’écran mettant en vedette Brad Pitt. Voici en vrac les morceaux présentés par O’Neil:
- Un modèle est un univers parallèle qui puise dans les probabilités, recensant tous les liens mesurables possibles entre les divers éléments d’un modèle (p. 16)
-
« Baseball has statistical rigor. Its gurus have an immense data set at hand, almost all of it directly related to the performance of players in the game. Moreover, their data is highly relevant to the outcomes they are trying to predict. This may sound obvious, but as we’ll see throughout this book, the folks using WMDs routinely lack data for the behaviors they’re most interested in. So they substitute stand-in data, or proxies. They draw statistical correlations between a person’s zip code or language pattern and her potential to pay back a loan or handle a job. These correlations are discriminatory, and some of them are illigal. » (p. 17-18)
- En ce qui concerne le baseball, « data is constantly pouring in. […] Statisticians can compare the results of these games to the predictions of their models, and they can see where they were wrong […] and tweak their model and […] whatever they learn, they can feed back into the model, refining it. That’s how trustworthy models operate » (p. 18)
-
« A model, after all, is nothing more than an abstract representation of some process, be it a baseball game, an oil company’s supply chain, a foreign government’s actions, or a movie theater’s attendance. Whether it’s running in a computer program or in our head, the model takes what we know and uses it to predict responses in various situations. » (p. 18)
- Mais, rien n’est parfait dans un monde de statistiques: « There would always be mistakes, however, because models are by their very nature, simplifications. » (p. 20) Ils ont des points morts (blind spots), certains éléments de la réalité qui ne modélisent pas ou des données qui ne sont pas incorporées dans le modèle. « After all, a key component of every model, whether formal or informal, is its definition of success. » (p. 21)
-
« The question, however, is whether we’ve eliminated human bias or simply camouflaged it with technology. The new recidivism models are complicated and mathematical. But embedded within these models are a host of assumptions, some of them prejudicial. And while [a convicted felon’s] words were transcribed for the record, which could later be read and challenged in court, the workings of the recidivism model are tucked away in algorithms, intelligible only to a tiny elite. (p. 25)
- Exemple du LSI-R: level of service inventory – revised, un long formulaire à être rempli par un prisonnier. (p. 25-26)
- Afin de cerner le concept d’armes de destruction mathématiques, O’Neil propose trois facteurs, présentés sous forme de questions, afin d’identifiers quels algorithmes se qualifient. La première question s’articule ainsi: « Even if the participant is aware of being modelled, or what the model is used for, is the model opaque, or even invisible? […] Opaque and invisible models are the rule, and clear ones very much the exception. » (p. 28) O’Neil cite la propriété intellectuelle, et son corollaire malsain, le secret industriel, à défaut d’une obligation de divulgation (comme les brevets – cette observation est mienne) comme étant la cause de cette opacité et de cette invisibilité. Ce qui introduit la seconde question: « Does the model work against the subject’s interest? In short, is it unfair? Does it damage or destroy lives? » (p. 29) Cette iniquité découle d’un système de rétroaction déficient (feedback loop). Finalement, la troisième question est « whether a model has the capacity to grow exponentially. As a statistician would put it, can it scale? […] the developing WMDs in human resources, health, and banking just to name a few, are quickly establishing broad norms that exert upon us something very close to the power of law. » (p. 29-30)
- « So to sum it up, these are the three elements of a WMD: Opacity, Scale, and Damage. […] And here’s one more thing about algorithms: they can leap from one field to the next, and they often do. » (p.31)
Pas besoin d’avoir un doctorat en droit pour comprendre que les choses aux États-Unis sont bien différentes qu’ailleurs : leurs droits à la vie privée ne sont pas enchâssés dans des constitutions ou édictés par des lois. Le recours aux méthodes statistiques (Monte Carlo) appliquées à des modèles ou à des jeux de données incomplètes perpétuent des préjugés et les érigent en systèmes étanches, inhumains, injustes.
Dans le reste de son livre, O’Neil nous peint un portrait glauque mais lucide de la dystopie algorithmique qui s’installe tranquillement aux USA. Les pauvres, les marginalisés ou les illettrés sont proie aux analyses des machines tandis que les fortunés sont analysés par des humains.
De tous les chapitres, celui sur la justice m’interpelle le plus.
Dans un premier temps, O’Neil présente l’algorithme prédictif employés par les services de polices afin de déterminer où se produiront les crimes, tels l’outil PredPol ou le projet CompStat de la ville de New York. O’Neil relate comment d’autres approches des forces de l’ordre, dont la méthode stop-and-frisk (qui consiste à interpeller quiconque semble le moindrement suspect), ne fait que renforcer le modèle d’oppression envers certaines communautés qui se concentrent dans des quartiers précis…
D’un point de vue plus large, les réflexions de O’Neil concernant les WMDs me fait penser aux théories de Shannon sur la communication ainsi que de Wiener sur la cybernétique, (liens vers des billets synthétiques sur ces théories). En particulier, le cadre d’analyse de O’Neil (ses trois questions ci-haut, pour déterminer si un algorithme est un WMD) évoquent les trois éléments de Shannon et Wiener pour l’information: communication, rétroaction, entropie.
J’ai aussi découvert un autre livre potentiellement intéressant: Unfair : the new science of criminal injustice par Adam Benforado chez Crown Publishers en 2016. Je me suis empressé de l’emprunter pour creuser cette fâcheuse intersection entre le droit et les mathématiques…
Je viens de jeter un coup d’oeil au livre de Benforado. Quoi que bien écrit et à première vue une critique constructive du système judiciaire, je ne vais pas effectuer une lecture plus approfondie. En fait, il traite spécifiquement des développements en psychologie cognitive, comportementale et en neuropsychologie (comment nous percevons le monde, comment nous nous expliquons nos biais personnels, comment notre cerveau réagit à des stimulus perçus consciemment ou non). De plus, il traite uniquement du système criminel et pénal américain, ce qui me semble bien loin de mes algorithmes et de mes mathématiques et le droit. Une bonne lecture donc, pour une autre fois.
Appel de communication Document numérique France
Études sur les doigts
Olivier Charbonneau 2014-10-29
J’ai souvent des conversations intéressantes avec des collègues français au sujet du zèle que nous québécois portons à maintenir l’intégrité linguistique de la langue française. Il trouvait que l’on exagère, il faut laisser une langue vivre, respirer, se développer. Peut-être, mais en tant que simple blogueur francophone sur les sujets techno-juridiques, je dois avouer que je suis témoin de belles bourdes et autres laxismes terminologiques.
Un anglicisme est inacceptable lorsqu’un mot valide existe déjà. Un néologisme est pertinent lorsqu’une idée ne s’exprime pas convenablement dans notre langue et il est convenable de maintenir un certain décorum, une intégrité phonético-poétique.
Je reçois dans mes courriel d’une autre collègue bien intentionnée une note concernant un appel de communications pour le lancement d’une nouvelle revue scientifique, publiée chez Classiques Garnier, intitulée: Études digitales.
Et hop. Je lance l’application du Petit Robert sur mon portable et je vérifie une source autoritaire sur la question. Digital réfère aux doigts. Il faut dire numériques, sinon nous employons un anglicisme.
Je croyais un canular – honnêtement, le site de l’éditeur ne mentionne pas encore la revue. Et l’appel concerne l’écriture de texte, alors il y a quand même un lien avec les doigts car ces appendices servent de vecteur aux mots numériques.
En vertu des dispositions sur l’utilisation équitable (art. 29 et suivantes) de la Loi canadienne sur le droit d’auteur, je vous communique l’appel de textes de cette revue sur le bout des doigts:
Appel pour le numéro 1 de la revue Études Digitales
« Le texte à venir »
La revue sera publiée chez Classiques Garnier
Le numéro 1 de la revue Études Digitales sollicite l’ensemble des humanités pour tenter d’appréhender les enjeux d’une mutation du texte par l’effet de sa digitalisation. Qu’est-ce que le texte digital ? Après le texte manuscrit, imprimé ou « tapuscrit » à la machine, le texte écrit au clavier d’ordinateur ou sur une tablette doit-il être envisagé comme la poursuite d’une même tradition textuelle ou au contraire ne vient-il pas produire un nouvel « encodage » ? Après le texte relié et édité sous la forme du codex, le texte à l’écran produit-il les mêmes effets ? Le texte digital est-il texte au sens des régimes antérieurs de texte, ou est-il résolument d’une autre nature ? N’assistons-nous pas à une transformation de sa grammatisation en même temps que se modifient les techniques de sa reproduction et de sa diffusion comme cela s’est produit au XVe siècle avec l’avènement de l’imprimerie ?
Plusieurs dimensions du texte digital seront envisagées dans ce numéro 1 : sa matérialité à travers les appareils et les interfaces qui le rendent visible, son mode de conservation et d’organisation de la mémoire, son inscription paradigmatique dans les représentations du savoir, les pratiques qu’il permet dans un contexte interopérable. Seront également traités les échanges qu’il autorise, les formes d’interprétation qu’il suscite ou facilite, plus largement, les questions qu’il pose à une culture fondée sur la conservation des textes, en termes d’historicité et d’invention poétique.
Le texte digital, de par son caractère dématérialisé et hypertextuel, ne peut être considéré comme le simple transfert du texte imprimé sur un nouveau support. Il produit des effets inédits dans le monde de l’écrit : multiplicité des traces jusqu’à l’hypermnésie, duplication indéfinie, mais aussi annulation et effacement, perte ou dilution de la source au profit d’une auctoritas partagée.
Genette définit la transtextualité comme « tout ce qui met (le texte) en relation, manifeste ou secrète, avec d’autres textes ». Ceci permet d’envisager une transtextualité digitale qui met en œuvre, par sa constitution même, un mode général de transversalité et d’hybridation. Ainsi, le numéro 1 de la revue Études Digitales s’ouvre-t-il à une conception élargie du texte, ainsi qu’à tout ce qui se situe au-dessus, au-dessous et autour du texte.
Plusieurs dimensions de la textualité digitale pourront être investies :
Le texte digital entre production, commentaire et invention
L’évolution du support suscite de nouvelles formes d’écriture et de lecture, mais surtout un texte digital ne peut être envisagé selon les mêmes critères qu’un texte imprimé. Il s’agit d’un objet hybride et composite dont les contours ne s’arrêtent pas aux limites de la page ou du volume qui constituaient la forme des textes depuis le codex antique. Un texte digital peut se trouver relié à une multitude d’autres textes (hypertexte) et conserver, comme un wiki, toutes les strates de sa production. Comment produire le texte digital ? Comment envisager une fabrication qui ne soit pas simplement le transfert des pratiques antérieures d’écriture ? Faut-il envisager une herméneutique spécifique du texte digital parallèlement à l’émergence d’une poétique qui rapprocherait le commentaire de l’invention ? Devons-nous en attendre de nouveaux genres, une nouvelle littérature ?
Le texte digital et les savoirs
À l’intérieur de la tradition occidentale, chez Platon, la fixation textuelle apparaît comme un enjeu du savoir, de sa transmission et son organisation. Aujourd’hui, le texte digital réorganise la disposition et le classement des savoirs inscrits, produisant de nouvelles modalités d’accès, de représentation. Faut-il voir dans l’écriture digitale une textualité débarrassée des limites anciennes du texte imprimé et relié et un nouvel espace du texte « à venir » ? Des modes d’élaboration contributifs construisent-ils une articulation pertinente des échanges entre les spécialistes et les amateurs ? Quel format de savoir instruit le texte digital ?
Le texte digital entre mémoire générale et oubli
Dans le contexte digital, les régimes d’une mémoire, à la fois hypermnésique et instable, se trouvent soumis à l’obsolescence des supports et des formats. Quel est alors le statut mémoriel du texte ? Quels seront les modes de conservation et d’indexation du texte digital ? Comment retrouver l’état génétique d’un texte pour le replacer dans l’archéologie des disciplines, quand archive et trace deviennent problématiques ?
Direction de la revue : Franck Cormerais & Jacques Athanase Gilbert
Comité éditorial : Antonioli Manola (Ecole Supérieure d’Art et de Design de Valenciennes) – Catellin Sylvie (Université Versailles Saint-Quentin) – Komur Greta (Université de Mulhouse) – Le Deuff Olivier (Bordeaux-Montaigne) – Lefevre Anne (École Supérieure d’Architecture de Saint Etienne) – Loty Laurent (Paris IV, CNRS) – Marechaux Pierre (Université de Nantes) – Noyer Jean-Max (Université de Nice) – Puig Vincent ( IRI, Centre Pompidou) – Rey Olivier (CNRS) – Rousseaux Francis (Université de Reims/IRCAM) – Vitali-Rosati Marcello (Université de Montréal) –
Comité scientifique: Bachimont Bruno (Université de Compiègne) – Philippe Béraud (Télécom Bretagne) – Bouchardon Serge (Université de Compiègne) – Clivaz Claire (Université de Lausanne) – Carayol Valérie (Bordeaux-Montaigne) – Duféal Marina (Université de Bordeaux-Montaigne) – Giffard Alain (Administrateur civil/Fondateur de Gallica) – Ertzscheid Olivier (Université de Nantes) – Galinon-Mélénec Béatrice (Normandie Université-ULH) – Gefen Alexandre (Paris IV – Fabula) – Jauréguiberry Francis (Université de Pau, CNRS) – Khatchatourov Armen (Télécom ParisTech) – Kerouanton Jean-Louis (Université de Nantes) – Lescop Laurent (ENSAM Nantes) – Moore Gerald (Durham University) – Musso Pierre (Rennes2/ParisTech) – Prié Yannick (Université de Nantes) – Proulx Serge (UQAM Montréal) – Romele Alberto (Université de Porto) – Stiegler Bernard (Conseil National Supérieur du Numérique/Université de Londres/Goldsmith) – Vignon Daphné (Université de Nantes)
Projet d’article pour le 30 novembre 2014 d’environ 3000 signes (sans les espaces). La réponse d’acceptation sera donnée avant la fin décembre. Les articles seront à rendre pour le 1er février, ils seront soumis à la lecture en double aveugle des membres du comité scientifique. Longueur des articles : 30000 à 35000 signes (sans les espaces et bibliographie comprise).
Envoyer les propositions et articles à : etudesdigitales@gmail.com
Franck Cormerais et Jacques Athanase Gilbert
Franck.cormerais@u-bordeaux3.fr
jacques.gilbert@univ-nantes.fr
Ils sont fous ces Français !